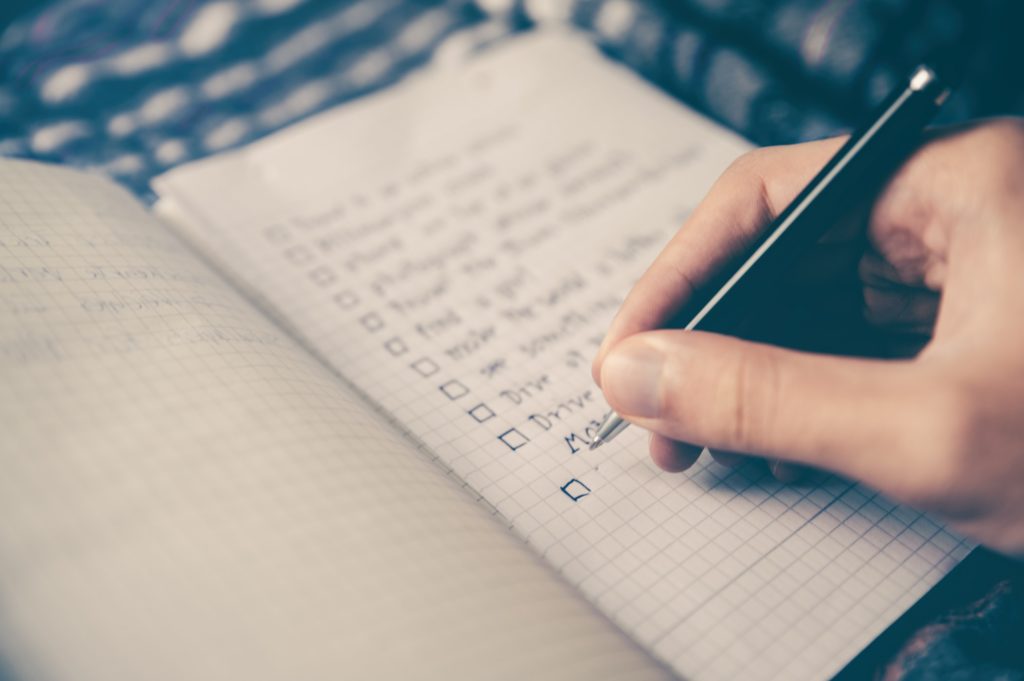
According to the Insurance Information Institute, insurance fraud accounts for nearly 10% of the property and casualty insurance industry’s incurred losses and loss adjustment expenses each year. Ten percent is a stunningly large loss driver that is often under-captured, and it hurts carriers’ bottom line by billions of dollars every year.
Looking on the bright side, the insurers who invest in reducing fraud effectively and improving risk selection and pricing will ultimately improve their loss ratio.
Fraud detection is an arms race, where insurers need to identify and block avenues for fraudulent claims as fast as fraudsters can create them. Here’s how insurance companies can fight back against fraud.
How is insurance fraud detected?
One of the most common anti-fraud technologies used today by insurance companies is a rules-based system.
Rules-based systems serve a basic purpose of catching obvious fraud patterns with a “black and white” logic. The approach is generally not very effective at uncovering new and novel schemes or adapting to emerging fraud patterns or schemes. As a result, insurers’ existing fraud programs are vulnerable to many types of fraud that aren’t obvious.
How are insurance companies improving their fraud detection?
Some insurance companies understand the limitations of a rules-based approach and are increasingly turning to more sophisticated anti-fraud technologies such as predictive modeling, link analysis, and artificial intelligence.
Insurers should carefully evaluate fraud detection techniques and technologies to make the most informed decision. Generally, using machine learning models as a standalone solution or complement to existing rules-based systems is advantageous.
3 Key Factors to Consider in a Machine Learning Model
1. Make sure your fraud models scale.
Your rules library must keep expanding and adjusting as fraud evolves. In short, you need to make sure your anti-fraud technology has scalability.
A rules-based approach can make the system slower and puts a heavy maintenance burden and cost on your team of fraud analysts, requiring an increasing number of manual reviews.
Machine learning requires minimal human involvement as the models learn automatically from both old and new data.
Machine learning algorithms and models become more effective with increasing data sets. Contrast this with rules-based models where the cost of maintaining the fraud detection system multiplies as claims grow. Machine learning is more efficient, as it improves with more data and can pick out the subtle differences and similarities between multiple data variables.
2. Models should have expansive detection coverage and accuracy.
Rules-based fraud detection systems can be too broad. They run a high risk of false positives. This reduces investigator efficiency and can lead to a negative customer experience when a smooth and empathetic customer experience is most vital. To prevent this, insurers often change the rules to capture only the claims with the highest probability of fraud. This is a flawed approach, as vast numbers of fraudulent claims can slip by and be paid out.
When special investigative units or other claims staff verify and self-report the claims they think are worthy of further investigation, machine learning models can learn and get better at predictions over time.
Machine learning-based fraud systems can be more accurate, more configurable, and easier to improve than rules-based systems. This makes fraud analysis easier and more effective, especially to keep up with all of the latest scams or fraud methods.
3. Models should optimize for efficiency.
Implementing if-then rules for a rules-based approach is easy at first, but it requires manual work and supervision to maintain. The result is a technology that doesn’t adapt well over time. It can be challenging to find the exact rule you might wish to change, and repeating this process each time, can be frustrating and time-intensive.
Machine learning can evaluate many claims fast and in real-time because it continuously analyzes and processes new data. You can set up machine learning from first notice of loss to claims closure.
Moreover, advanced machine learning models such as neural networks can autonomously update their models to reflect the latest trends and prior fraud results. The system also enables a more flexible and automated approach that speeds up fraud management and conversion optimization.
The bottom line
Machine learning-based fraud systems enable the automatic detection of known and unknown fraudulent claims. It can analyze patterns at the first notice of loss and throughout the claims process.
Unlike the rules-based systems, which only produce a yes or no response to fraud, machine learning produces a fraud suspicion score from 0 to 1000, like a credit score. It also provides context that allows insurance carriers to take different actions based on their different risk tolerances.
In short, machine learning offers a more personalized and adaptive approach. Insurance carriers can automate the passing of less-suspicious claims for faster claims payouts and flag more suspicious claims for further investigation. Machine learning empowers special investigative units, claims teams, and other fraud professionals to make more informed investigation decisions faster.
Metromile Enterprise provides insurance carriers with machine learning technology to combat fraud. Machine learning models are better at surfacing suspicious claims than the rules-based systems most insurers use today and have a higher chance of identifying fraud that isn’t as obvious. It can also be more accurate and scalable.
Whether used with existing rules-based approaches or on its own, machine learning-based fraud systems create net new opportunities for insurers to establish a robust fraud analytics program within their claims organization. The result is a nimble, cost-effective claims department that delivers strong loss and loss adjustment expense reductions.
To learn more about applying artificial intelligence and machine learning in your claims organization, contact us. A large P&C carrier established a robust fraud analytics program in less than six months with Metromile Enterprise.